AI Exhibits Deep-Seated Bias Against African American Vernacular English
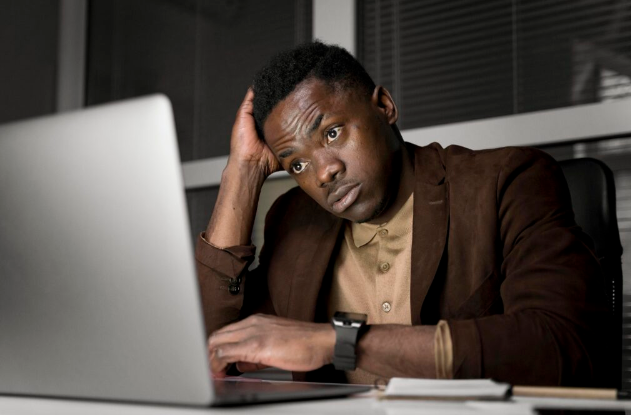
Recent studies have revealed that AI systems, particularly those used in natural language processing (NLP), exhibit significant bias against African American Vernacular English (AAVE). This bias raises concerns about the fairness and inclusivity of AI technologies that are increasingly integrated into everyday life.
Researchers have found that many AI models, including those used in voice recognition, text analysis, and automated decision-making, struggle to accurately understand and process AAVE. These models often misinterpret words and phrases used in AAVE, leading to higher error rates and, in some cases, the unfair treatment of individuals who speak this dialect.
The roots of this bias lie in how AI systems are trained. Most AI language models are trained on large datasets that predominantly feature Standard American English, with little to no representation of AAVE. As a result, these systems become proficient in recognizing and processing Standard English but fail to accurately handle the linguistic nuances of AAVE. This lack of diversity in training data leads to systemic disadvantages for speakers of AAVE when interacting with AI-driven technologies.
The implications of this bias are far-reaching. In the context of voice-activated assistants, job application screeners, or even automated customer service bots, the inability to properly understand AAVE can result in misunderstandings, missed opportunities, or unfair treatment. For example, in job recruitment, an AI system might incorrectly assess the communication skills of a candidate who speaks AAVE, potentially leading to biased hiring decisions.
Addressing this issue requires a concerted effort to diversify the data used to train AI models. Developers are being urged to include a wider range of linguistic and cultural representations to ensure that AI systems are more inclusive and equitable. Additionally, there is a growing call for more transparency in how these systems are trained and evaluated, so that biases can be identified and corrected.
As AI continues to play a larger role in society, ensuring that these systems are fair and unbiased is crucial. The bias against AAVE in AI systems is just one example of the broader challenges in making technology truly inclusive, but it serves as a critical reminder of the importance of diversity in the development of these powerful tools.