The Past and Future of AI (with Dwarkesh Patel)
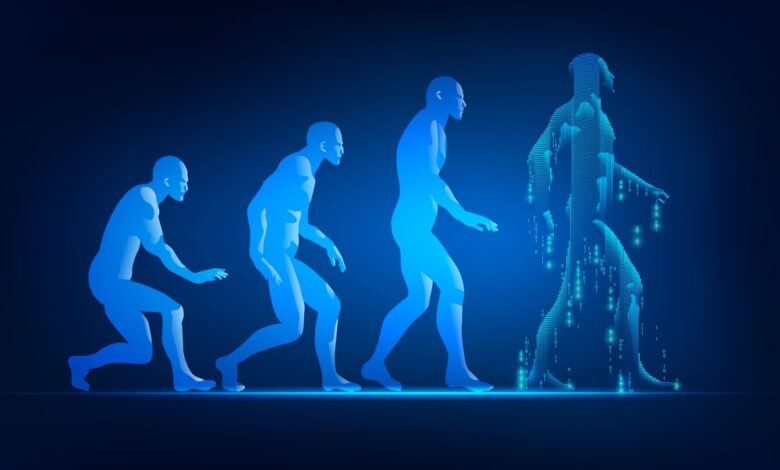
The conversation between Russ Roberts and Dwarkesh Patel delves into the intricacies of artificial intelligence and its evolution over the past six years. Dwarkesh Patel, a podcaster and author, along with Gavin Leech, sheds light on the significant trends that have shaped the AI landscape from 2019 to 2025.
One of the key points discussed is the exponential growth in compute power and data accumulation, which has paved the way for groundbreaking advancements in AI algorithms. Dwarkesh emphasizes the importance of understanding the role of compute power in driving innovation and the development of sophisticated AI models like the transformer.
The transformer, a revolutionary architecture introduced by Google researchers in 2018, has played a pivotal role in the evolution of AI models such as ChatGPT and large language models. Its parallel training capability and predictive learning process have enabled researchers to scale up AI models and achieve remarkable levels of intelligence.
Despite the impressive progress in AI technology, Dwarkesh highlights the limitations of pre-training models like GPT-4.5 and emphasizes the need to focus on inference scaling and task-specific training to unlock the full potential of AI. By training models to solve specific problems such as coding and math, researchers aim to tackle more complex and ambiguous tasks that require multiple consecutive steps.
The conversation also touches upon the challenges of AI automation in remote work scenarios, where models struggle to replicate human cognitive abilities and adaptability. While AI has made significant strides in certain domains, there is still much work to be done to enhance its problem-solving capabilities and real-world applications.
Overall, the discussion between Russ Roberts and Dwarkesh Patel offers valuable insights into the underlying trends and technologies driving the AI revolution. By reexamining the past six years of AI development, researchers and enthusiasts can gain a deeper understanding of the challenges and opportunities that lie ahead in the field of artificial intelligence. The conversation between Russ Roberts and Dwarkesh Patel sheds light on the current state of AI research and the potential future developments in the field. Russ reflects on his personal use of AI technology, particularly his admiration for Claude, an AI model he finds visually appealing and useful for various tasks such as brainstorming, tutoring, translation, and travel advice.
Dwarkesh, on the other hand, discusses his reliance on AI models for research and podcasting, highlighting their moderate usefulness in automating certain tasks. He poses an intriguing question about the limitations of current AI models in performing practical tasks such as booking flights or organizing logistics, despite their proficiency in solving complex math problems.
The conversation delves into the idea of “computer use” by AI models, where the ability to perform practical tasks akin to a high school student is still a challenge for these advanced systems. Dwarkesh raises the question of whether common-sense reasoning and practical problem-solving capabilities are intrinsic limitations of current AI models or areas that require further research and development.
The analogy of Deep Blue defeating Kasparov in chess is referenced, highlighting the milestone achieved in AI capabilities but also emphasizing the ongoing challenges in replicating human-like intelligence across various domains. The discussion hints at the potential for advancements in AI research to bridge the gap between complex problem-solving and everyday practical tasks, paving the way for more integrated and versatile AI applications in the future.
Overall, the conversation between Russ Roberts and Dwarkesh Patel offers valuable insights into the current landscape of AI research, the practical applications of AI models, and the evolving challenges and opportunities in the field. As AI technology continues to advance, the quest for achieving human-like intelligence and common-sense reasoning remains a key focus for researchers and developers in the pursuit of creating more sophisticated and useful AI systems. In looking back at the development of chess engines, it becomes apparent that they lack many essential components needed for tasks beyond playing chess. The narrow focus of these engines highlights the limitations of current AI technology in terms of automating complex tasks like coordinating workers.
Reflecting on this, one wonders if future advancements in AI will reveal the true potential of long-term agency, coherence, and common sense. Perhaps the current models are just scratching the surface of what AI can achieve.
During an interview with Dario Amodei of Anthropic, the discussion turned to the mystery of why scaling works in AI. Despite the massive amounts of data and compute power thrown at these models, the underlying explanation for their intelligence remains elusive. This uncertainty poses a significant hurdle in improving AI systems to perform tasks like booking trips or making decisions beyond providing information.
The challenge lies in enhancing AI models to not only offer advice but also take action in a way that mimics human intelligence. The gap between the current capabilities of AI and the desired level of functionality raises questions about how to bridge that divide.
One key question that remains unanswered is why AI models, with access to vast amounts of information, struggle to make creative connections and discoveries like humans do. While humans can draw upon their knowledge to come up with innovative solutions, AI models seem to fall short in utilizing their vast memory capacity for intelligent ends.
As we continue to explore the potential of AI, it is clear that there is much work to be done in understanding and enhancing the capabilities of these systems. With ongoing research and advancements in the field, we may soon unlock the full potential of AI in solving complex problems and achieving truly intelligent outcomes.